ST-MNIST
ST-MNIST - The Spiking Tactile-MNIST Neuromorphic Dataset
TL;DR
We debuted a neuromorphic tactile MNIST dataset, and provided its classification accuracies to serve as performance benchmarks for future work.
Tactile sensing is an essential modality for smart robots as it enables them to interact flexibly with physical objects in their environment. Recent advancements in electronic skins have led to the development of data-driven machine learning methods that exploit this important sensory modality. However, current datasets used to train such algorithms are limited to standard synchronous tactile sensors. There is a dearth of neuromorphic event-based tactile datasets, principally due to the scarcity of large-scale event-based tactile sensors. Having such datasets is crucial for the development and evaluation of new algorithms that process spatio-temporal event-based data.
In this work, we debut a novel neuromorphic Spiking Tactile MNIST (ST-MNIST) dataset. ST-MNIST comprises handwritten digits (0-9) generated by 23 human subjects writing on a 100-taxel biomimetic event-based tactile sensor array. We also describe an initial effort to evaluate our ST-MNIST dataset using existing artificial and spiking neural network models. The classification accuracies provided herein can serve as performance benchmarks for future work.
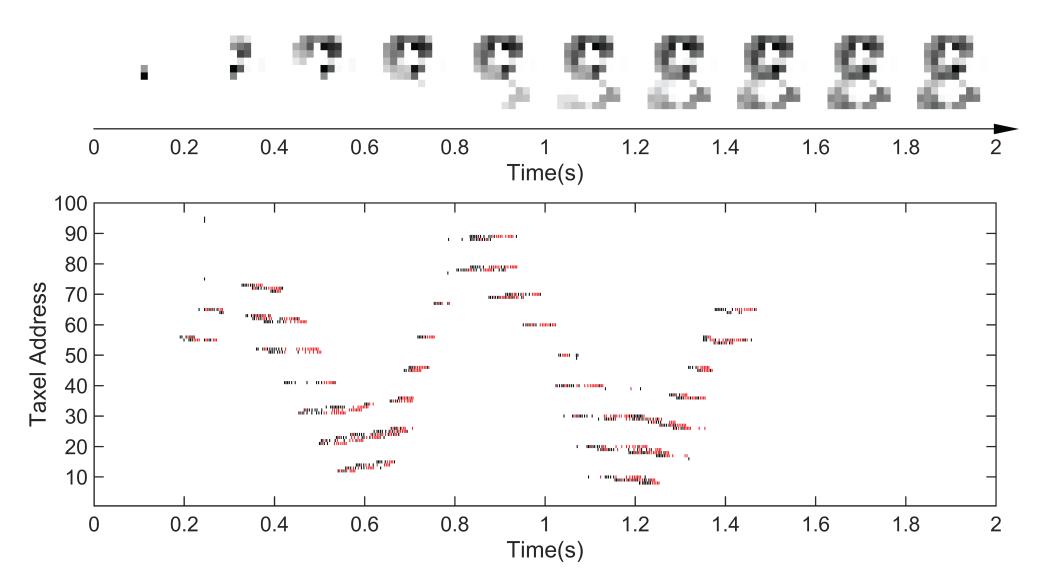
Resources
Citation
If you build upon our results and ideas, please use this citation.
---
@article{see2020st,
title={ST-MNIST--The Spiking Tactile MNIST Neuromorphic Dataset},
author={See, Hian Hian and Lim, Brian and Li, Si and Yao, Haicheng and Cheng, Wen and Soh, Harold and Tee, Benjamin CK},
journal={arXiv preprint arXiv:2005.04319},
year={2020}
}
---